SMILE-UHURA Challenge
Vessel Segmentation Challenge, at ISBI 2023
to be held in Cartagena de Indias, Columbia from 18th to 21st April 2023
Timeline and Contact Details
Current Phase: Registrations open, training data is to be releasedRegistration Opens: 02.01.2023
Release of the Training Dataset: 15.01.2023
Docker Submission Opens: 15.02.2023
Abstract Submission Opens: 20.02.2023
Abstract Submission Deadline: 15.03.2023
To participate, visit synapse.org/uhura
For any queries (except for technical issues related to Synapse or Docker), please send an email to uhura@soumick.com OR smileuhura@pm.me. For any technical assistance (including problems with Synapse or docker container), please contact Hannes Shnurre via email: hannes.schnurre@ovgu.de OR phone: +49 391 67-56116
SMILE-UHURA 2023
Small Vessel Segmentation at MesoscopIc ScaLE from Ultra-High ResolUtion 7T Magnetic Resonance Angiograms
The human brain receives nutrients and oxygen through blood vessels in the brain. Pathology of small vessels, i.e. mesoscopic scale, is a vulnerable component of the cerebral blood supply and can result in major complications such as Cerebral Small Vessel Diseases (CSVD). Higher spatial image resolution can be reached with the advancement of 7 Tesla MRI systems, allowing the visualisation of such vessels in the brain. This can be attributed to the fact that there is no public dataset available which provides high-resolution images acquired at 7T MRI along with annotated vessels. Using a combination of automated pre-segmentation and considerable manual refining, an annotated dataset of Time-of-Flight (ToF) angiography acquired with a 7T MRI was generated to create the foundation of this challenge. SMILE-UHURA provides this annotated dataset to train machine learning models and creates a platform to benchmark different approaches.
This challenge is co-located with SHINY-ICARUS: Segmentation over tHree dImensional rotational aNgiographY of Internal Carotid ArteRy with aneUrySm
How to participate?
This challenge will be hosted over Synapse. The interested participants are requested to visit synapse.org/uhura and register as soon as possible to join the team of participants. The training data will be made available over Synapse by the 15th of January 2023. The participants would need to use them to train their methods and create a docker container following the provided template. The docker container has to be uploaded to Synapse for evaluation. The participants need to become a Certified User on Synpase to be able to submit the their docker containers. For any technical assistance (including problems with Synapse or docker container), please contact Hannes Shnurre via email: hannes.schnurre@ovgu.de OR phone: +49 391 67-56116
For any queries (except for technical issues related to Synapse or Docker), please send an email to uhura@soumick.com OR smileuhura@pm.me
More details will be announced soon.
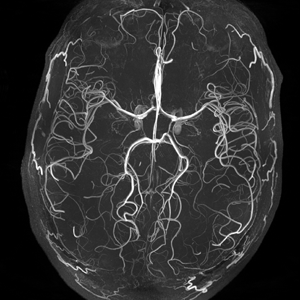
Organisers:
Dr Soumick Chatterjee
Postdoctoral Researcher, Genomics Research Centre, Fondazione Human Technopole, Milan, Italy &
Guest Researcher (PostDoc), Faculty of Computer Science, Otto von Guericke University Magdeburg, Germany
Dr Hendrik Mattern
Postdoctoral Researcher, Department of Biomedical Magnetic Resonance, Otto von Guericke University Magdeburg, Germany
Dr Florian Dubost
Machine Learning Engineer, Liminal Sciences, Inc., USA
Prof Dr Stefanie Schreiber
Group Leader & Senior Neurologist at the Department of Neurology, Medical Faculty, University Hospital of Magdeburg, Germany
Prof Dr Andreas Nürnberger
Professor, Institute of Technical and Business Information Systems, Faculty of Computer Science, Otto von Guericke University Magdeburg, Germany
Prof Dr Oliver Speck
Professor, Institute for Physics, Faculty of Natural Sciences, Otto von Guericke University Magdeburg, Germany
Brain function relies on the cerebral vasculature to supply nutrients and oxygen. Therefore, any impairment of the vasculature can damage the brain tissue, which can lead to cognitive decline. The cerebral vasculature is organised as a hierarchical, tree-like network; hence, with increasing branch order, the vessel diameter decreases and the number of vessel branches increases.
While for the major cerebral vessels, i.e. macroscopic scale, and for the capillaries, arterioles, and venules, i.e. microscopic scale, in vivo and ex vivo imaging modalities exist, respectively, assessment of the mesoscopic scale (vessel diameter of 100-500µm) is challenging. However, mesoscopic vessel pathology can be associated with ageing, dementia and Alzheimer's disease and Segmentation-quantification of these vessels is an important step in the investigation of Cerebral Small Vessel Disease (CSVD).
In recent years, ultra-high field (UHF) magnetic resonance imaging (MRI) has been used to bridge macroscopic and microscopic assessment of the human cerebral vasculature. Since the pioneering work on the magnetic resonance angiography (MRA) at 7 Tesla (7T), the imaging field has come a long way, acquiring the highest resolutions to date - presenting resolutions as high as 150 and 140 µm, respectively. With an ultra-high resolution, the mesoscopic vessels can be imaged. Although these vessels are highly relevant in cerebral small vessel diseases, neurodegeneration, as well as understanding the signal origin of the functional fMRI signal, automatic vessel segmentation at the mesoscopic scale is not established.
Motivated by the demand from the neurological and neuroscientific community, this challenge was initiated, focusing on the segmentation of the vasculature at the mesoscopic scale. Although vessel segmentation challenges have a considerable tradition in the field, working with UHF MRI at the mesoscopic scale has unique challenges compared to 2D microscopic or 3D macroscopic vessel imaging and segmentation: (i) Instead of a single 2D image per sample, a 3D volume is acquired, increasing the computational demand and rendering manual segmentation tremendously more time-consuming; (ii) Compared to macroscopic segmentation, the ultra-high resolution data is considerably noisier and vessel-to-background contrast poorer, rendering automatic and manual segmentation non-trivial.
These challenges have hindered the establishment of openly available data repositories and, therefore, high-performance mesoscopic vessel segmentation algorithms. There is no high-resolution 7T dataset with annotation available that can be used to train automatic machine learning based segmentation methods or to benchmark the performances of the methods for this task. To that end, an annotated dataset of Time-of-Flight (ToF) angiography acquired with a 7T MRI has been created using a combination of automatic pre-segmentation and extensive manual refinement. This dataset will be the basis of this challenge and can function as a benchmark for quantitative performance assessment in the future.
Benchmarking datasets and challenges focusing on vessel segmentations have been established in the past, like the DRIVE challenge for segmenting blood vessels from retinal images and lung vessel segmentation from computed tomography (CT) images that was hosted at ISBI. When it comes to vessel segmentations from MRA-TOF, there has not been any public challenge or open dataset available for benchmarking. There have been, however, other tasks with MRA-TOFs, like the ADAM challenge about microaneurysms and the VALDO challenge, Vascular lesion detection and segmentation - focusing on cerebral microbleeds, along with Enlarged perivascular spaces (EPVS), both were hosted at MICCAI. These challenges came with different tasks for MRA-TOF data, providing labels for that particular task. There are also public datasets like IXI, which provides a large MRA-ToF dataset. Even though there are public datasets MRA-ToF datasets available publicly, they are all acquired with MRI scanners with field strengths 1T, 1.5T, or 3T - and not with UHF scanners like 7T. Images obtained with high spatial resolution on a 7T MR scanner contain more small vessels in comparison to a 3T MR scanner. Furthermore, these datasets do not provide any annotations for vessels that can be leveraged for training automatic vessel segmentation approaches.
Current approaches for vessel segmentation: One of the most prevalent vessel enhancement algorithms is the Hessian-based Frangi vesselness filter, which is generally paired with a subsequent, empirically calibrated thresholding to get the final segmentation. This method's multi-scale properties allow for small vessel segmentation, however significant parameter fine-tuning may be necessary to achieve good sensitivity towards such vessels of interest. Canero and Radeva developed a vesselness enhancement diffusion (VED) filter that combines the Frangi filter with an anisotropic diffusion scheme, which was then further extended by constraining the smoothness of the tensor/vessel response function. A multi-scale Frangi diffusion filter (MSFDF) pipeline has been proposed recently to segment cerebral vessels from susceptibility-weighted imaging (SWI) and TOF‐MRA datasets that initially pre-selects voxels as vessels or non-vessels by performing a binary classification using a Bayesian Gaussian mixture, and then applies Frangi and VED filters. To achieve the best results, the aforementioned approaches need manual fine-tuning of the parameters for each dataset, or even for each volume. Furthermore, these methods need various pre-processing procedures, such as bias field correction, making pipeline execution time-consuming. In recent times, methods relying on different deep learning models have been widely proposed for different tasks, including vessel segmentation. One of the most popular deep learning models for different segmentation tasks, the UNet model, has also been applied for vessel segmentation in X-ray coronary angiography and in TOF-MRA images of patients with cerebrovascular disease. Furthermore, UNet-backed semi-supervised learning has also been successfully applied for blood vessel segmentation in retinal images, as well as in 7T MRA-ToF images. Even though deep learning method has been proposed for vessel segmentation in 7T MRA-ToF images, the study only relies on semi-automatically created noisy training labels. An openly available 7T MRA-ToF dataset for vessel segmentation (i.e. dataset that includes perfect annotations for the vessels) would enable researchers to develop further such automatic techniques and also would help future researchers to benchmark their approaches against the state-of-the-art.
The challenge will include two different datasets - both acquired at 7T MRI with an isotropic resolution of 300 μm. To put the resolution in the context of the other datasets, the IXI dataset contains images with a resolution of 450 μm, and the earlier research paper that performed vessel segmentation in 7T MRA-ToF used a resolution of 600 μm. The images of the first dataset, which will be used as the public dataset (for training, validation, and testing), were collected from the StudyForrest project, containing MRA-ToF at 300 μm from 20 healthy subjects. The second dataset also contains 7T MRA-ToF of 11 subjects at the same resolution and will be kept private as the "secret" test set for the actual evaluation. Additionally, the secret test dataset contains one additional volume from a different subject with 150 μm resolution - which will be used for additional assessment as well - to evaluate the generalisability of the method in terms of resolution.
Both datasets were annotated following a 3-step approach. Initially, a rough segmentation was obtained by thresholding in 3D Slicer. The segmentation was empirically tuned for each volume to create an initial binary mask with as little noise as possible. With this step, the majority of medium to large-scale vessels were segmented properly, while many of the highly relevant small vessels were not segmented. Then extensive manual fine-tuning of these segmentations was performed (i.e. removing noise and delineating the missing small vessels). Then finally, the annotations were verified by a senior neurologist. Additionally, ten plausible segmentations for each of the volumes were created in a semi-automatic fashion by manipulating the parameters of the Frangi filter and will also be made available. Additional annotations created using OMELETTE - an automatic small vessel segmentation pipeline will also be provided. All these different annotations can be used for benchmarking purposes or can also be used for trainings demanding multiple annotations (e.g. Probabilistic UNet).
Two of the biggest challenges in segmenting vessels in such high-resolution scans are segmenting the small vessels (i.e. apparent vessel diameter of 1-2 voxels) and maintaining the vessel continuity. It might be possible to address these problems by manually fine-tuning semi-automatic methods, but that is very time consuming and not scalable. The problem is further increased when it comes to high-resolution 3D volumes instead of a single 2D image per sample (like in the case of fundus images) - increasing the computational demand and rendering manual segmentation tremendously more time-consuming. Moreover, compared to images in macroscopic segmentation tasks, the ultra-high resolution data is considerably noisier and vessel-to-background contrast poorer, rendering automatic and manual segmentation non-trivial.
Data Policy: Both studies under which the data were acquired were approved by local institutional review board (IRB). The original volumes of the StudyForrest is already openly published, and the annotations of this dataset will be made and kept publicly available. Future researchers can also use this data in their research without restrictions, as long as the challenge paper is cited. The secret test set will always be kept private - for evaluation purposes during the challenge, and afterwards for future evaluations.
DS6 is one of the recently published works which focuses on the problem of vessel segmentation at the mesoscopic scale. Moreover, two more approaches for vessel segmentation from us, one focusing on vessel continuity and the other one focusing on improving the probabilistic prediction, will be published by the end of November. These two methods will also be used as baselines. Codes for all these baselines are (and will be) available publicly on GitHub.
There will two different levels of evaluation - on the public test set (which can also be performed by the participants on their own) and on the secret test set. The evaluation of the segmentation quality using similarity measures - Dice, Jaccard, Volumetric Similarity, and Mutual Information, as well as using a distance measure - Balanced Average Hausdorff Distance. The winners will be chosen based on who wins on the most number of metrics ($n$ out of five). Individual metric-wise results, along with that - results on different other metrics, will also be published. There will be different focus areas of the evaluation - overall segmentation performance, performance on small vessels, quality of vessel continuity. The first two evaluations will be performed quantitatively and the third one regarding vessel continuity will be evaluated qualitatively by a senior neurologist.
The challenge aims at creating an opportunity for researchers for bench-marking their automatic segmentation approaches for the task of vessel segmentation on high-resolution 7T MRI. The final results of the challenge will be submitted to Medical Image Analysis (MedIA) journal of Elsevier, which will include all the participants as co-authors.
The segmentation approaches developed during this challenge might also be possible to be used for other segmentation tasks, such segmentations of EPVS and cerebral microbleeds. Moreover, as very few labelled 7T datasets are publicly-available, and none for vessel segmentation, this challenge would create a benchmarking dataset for future researchers working with the vessel segmentation problem.